How Fairmarkit is using machine learning to optimize procurement
For many years, traditional procurement was based on the institutional knowledge of individual buyers who were experts in their particular field, and as a result, established strong personal relationships with vendors in their area.
The rise of Google and search engines expanded buyer vendor networks drastically. Buyers now have the ability to search for specific items and source them from various suppliers. However, the challenge still remains: finding new suppliers is a manual, time-consuming process. Additionally, for enterprise companies, procurement teams continue to struggle with the lengthy, cumbersome process of onboarding new vendors. Even if a buyer identifies a new potential vendor offering a lower price, sometimes the process of onboarding this vendor outweighs the benefit of cost-savings.
So how are procurement teams at enterprise companies expected to optimize nonstrategic spend and maximize budget if the process of onboarding new vendors is too unmanageable?
Machine learning (ML)
Before we dive into the specifics about the Fairmarkit platform, it's important to have a basic understanding of machine learning.
Machine learning is a category of computing in which a system develops the ability to learn, detect and reproduce patterns or behaviors without explicit coding. ML usually requires development of algorithms using advanced numerical and statistical techniques on curated datasets of interest. These algorithms are then employed to perform tasks such as providing recommendations, predictions or decisions. Another feature of ML algorithms is that they can be designed to continually learn from new data sources and/or feedback. That could be as simple as a user response to “Was this recommendation helpful?” or more nuanced, such as “How many incorrect product codes were identified correctly?”
Machine learning and the Fairmarkit platform
Fairmarkit optimizes tail spend using machine learning for sourcing, vendor identification, and automated quoting to accelerate the bid-to-buy process. The search recommendation engine is powered by machine learning and collects data about your previous buying experiences across the company and recommends vendors or suppliers to you based on multiple data points. These data points include specific buyer, department, sourcing category, vendor, location, and time of year.
The recommendation system also uses external data from vendor websites and catalogs across our Marketplace to provide additional recommendations. The engine can then recommend new vendors from the broader Fairmarkit vendor ecosystem, as well as identify existing registered vendors that are already part of your purchasing process, that you may not have known as able to provide a particular good or service.
Let’s say you enter a product description into Fairmarkit, just as you would into Google. The engine will automatically categorize the item using our machine learning algorithm which leverages fuzzy matching to classify even vague item or service descriptions. This process can use part numbers and manufacturer information, but it is not necessary to have that structured data—we can do this with just a description of the item.
Let’s walk through an example to help visualize how the Fairmarkit machine learning platform works.
In the image below, the item the buyer is looking to source is described as “PowerBroker Password Safe.” That is the only information we have to use, which is a clear but inexact description of the item. Note we do not have a manufacturer or part number to include either.

Using our machine learning algorithm, Fairmarkit provides a list of recommended vendors who were identified as supplying items that fall into the same UNSPSC category in previous transactions. These UNSPSC numbers are identified by our machine learning that aligns free text description to UNSPCS numbers. In this case, it is category 43232900, Information Technology Broadcasting and Telecommunication > Software > Networking Software.
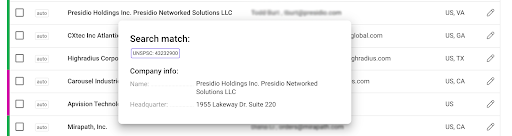
Predicting the most relevant vendors for a particular item and category
The vendor search result list consists of two main parts. Firstly, vendors who can supply these goods or services, and secondly, their relative rank, which factor in several variables. In order to rank the vendors appropriately, we need to take into account a lot of information. To predict the most relevant vendor, we have built a recommendation system utilizing a broad range of data points.
For the good or service up for bid, we take into account information like the category of the item(s), the number of items requested, the request maximum delivery time, the average delivery time for previous requests, the average item price, and the items’/services’ presence in vendor catalog data.
All of these metrics, along with previous historical transaction data and Fairmarkit’s collective intelligence, gives us our very specific set of vendors to recommend that should meet the buyer’s request for the good or service. In addition, during the sourcing process, diverse, local or preferred vendors can be tagged and presented with increased visibility. This saves buyers an enormous amount of time, eliminating monotonous, time-consuming tasks and streamlines the sourcing process.
What’s coming next? Our machine learning roadmap:
Our recommendation system is constantly updated based on the daily usage of the platform.
The goal is to provide procurement teams with a platform that utilizes available data to help buyers make more educated decisions, saving time for more strategic and meaningful sourcing events.
Here is a sneak peek of what is coming next on the Fairmarkit roadmap, as it relates to machine learning:
- Attachment recognition for more complex sourcing events
- Utilizing more context to classify items and identify vendors when an inexact product description is used
- Predicting the vendors that should be awarded—based on multiple metrics like price, trust, delivery time, shipping cost, etc.—and then validating those predictions against buyers’ real vendor selections
Interested in learning more?
Fairmarkit helps procurement teams accelerate the bid-to-buy process through streamlined sourcing, vendor identification, and automated quoting. Our solution uses machine learning and artificial intelligence to reduce manual effort by 30-40% on purchases while yielding savings of over 15%.
Set up time with one of our product specialists here and learn how you could save over 15% on all of your nonstrategic spend.